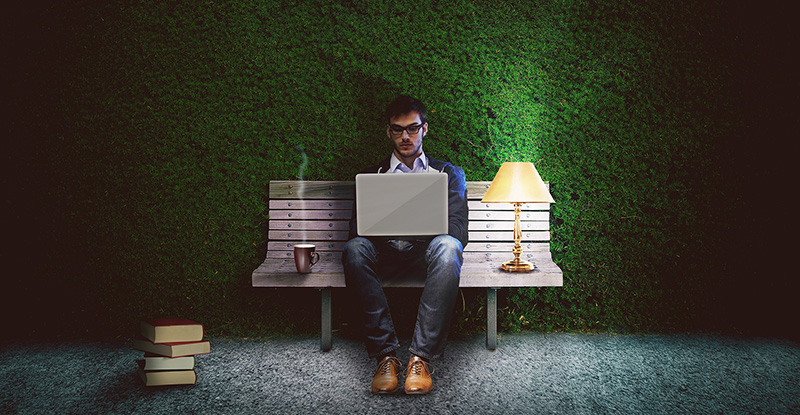
This two-part mini-series will introduce practical, and time-saving study tips split into two parts. The posts will focus on the tactics, that is using a larger goal to work backwards from, followed by tools, the daily tasks we complete to work towards that larger goal.
Why am I studying on a Wednesday night after a gruelling day at work?
First, define your long-term goals, then you can start broadly with your “why” to develop your strategy. Time management tools are essential to keeping yourself on track. Make sure you plan your studies so that you don’t find yourself in a jam, struggling to keep up with your personal and professional commitments.
Treat assignments as exam preparation.
CPA PEP module content covers the same competencies as the module and Common Final Exam. Often candidates see completing module tasks and studying for the exam as separate activities rather than complementary ones. Start with the end in mind by seeing each module assignment as exam preparation.
Follow this process as a framework to create your study plan for every module exam and the common final exam. Be sure to create your study plan early so you can take full advantage of it.
Write each practice case as if it were the exam.
Full stop. Establish these habits early on, and it will get easier even though screams of inner self-doubt will protest otherwise. I promise it will get easier IF you write all of your practice cases in exam-like conditions.
Debrief after each practice case.
Not debriefing is like permitting yourself to repeat the same mistakes without ever learning from them. It is effectively throwing minutes, hours, and days of studying away. Yes, it sucks to revisit a PC you just tried your best at, only to be faced with your shortcomings. Would you rather suspect you did not attain Competent by not debriefing a practice case, or would you rather have it confirmed by failing your module exam or CFE?
In my opinion, there is no option. Face your fears, head-on. Use the time you have saved by writing your practice case in the actual time provided to debrief properly. Refer to the learning eBook for tools on how to effectively debrief, and ensure you utilize feedback to fast-track your personal growth.
Remember, it’s not what you know when you submit your PC, but rather what you know when you debrief.
Get some help.
Your online facilitator is your first resource who will coach and guide you through each CPA PEP module, from Core 1 through Capstone 1. Capstone 2 candidates will receive marked PC feedback from CPA Canada’s National Marking Centre, where candidates may reach out to marking centre team leads directly via the candidate hotline. All CPA PEP modules have a regional discussion board for candidates to work with Lead Facilitators and each other toward material mastery.
If you’d like to explore additional resources, you can consider hiring a tutor or contacting CPAWSB’s Learner Support team: [email protected].
Do you have feedback on this post or a question you’d like answered by an experienced CPAWSB educator? Please contact your facilitator or send a question to the General Topic in the Candidate Discussion forum.
Samantha Taylor, PME, CPA, CA, is an educator and lead policy advisor for CPAWSB, and an instructor of accounting at Dalhousie University. She is on a mission to understand and enable learner efficacy while eliminating doldrums occasionally associated with accounting education. Read more of Sam’s posts at the CPAWSB blog.