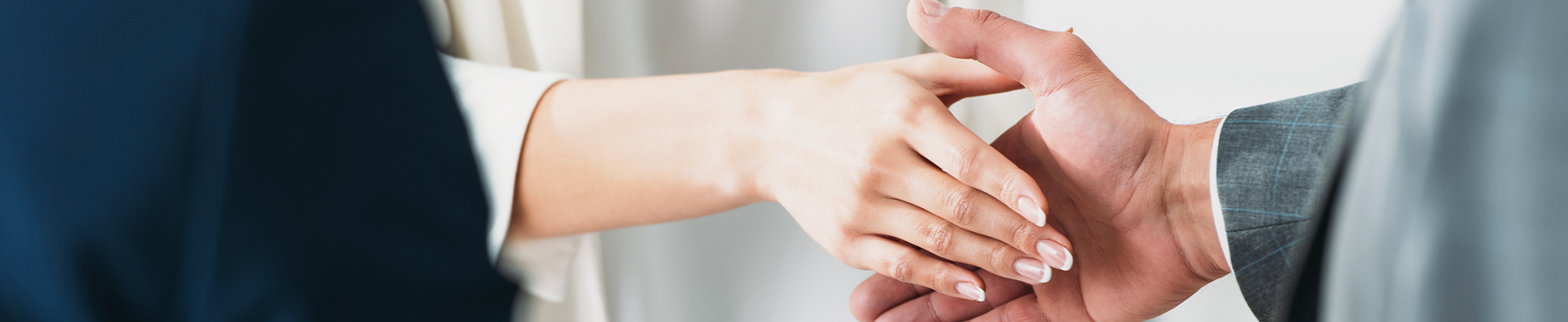
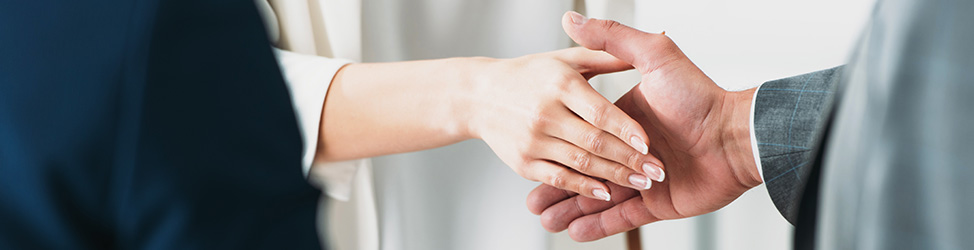
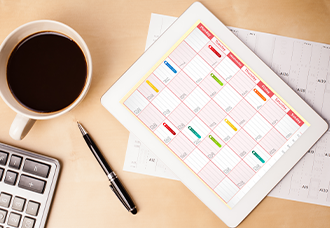
CPA PEP Schedule
The registration period closes at 11:59PM Mountain Time. Registrations received after this time are treated as a waitlist request. Waitlist requests will be accepted until one week before the module start date.
Candidates are required to submit a CPA PEP application before registering for Core 1. CPA PEP applications will be accepted until one month before the start of Core 1.
To complete CPA PEP prerequisites, please check out the CPA preparatory courses schedules. CPA preparatory courses are designed for those who lack some or all of the prerequisite knowledge required for admission to the (CPA PEP).
CPA PEP Sessions
Extended Winter 2025
Session | Registration Period | Module Dates | Withdrawal Deadline | Orientation Workshop | Module Workshop | Exam Date | Results Release | Appeals Deadline | Register |
---|---|---|---|---|---|---|---|---|---|
Core 1 | Oct 14 to Nov 25 | Jan 25 to Jul 3 | Feb 21 | Jan 25 to Jan 26 | Apr 26 to Apr 27 | Jul 3 (Thursday) |
Jul 18 | Jul 22 | Closed |
Core 2 | Oct 14 to Nov 25 | Jan 25 to Jul 4 | Feb 21 | N/A | May 10 to May 11 | Jul 4 (Friday) |
Jul 18 | Jul 22 | Closed |
Performance Management | Oct 14 to Nov 25 | Jan 25 to Jul 3 | Feb 21 | N/A | Feb 8 to Feb 9 | Jul 3 (Thursday) |
Jul 18 | Jul 22 | Closed |
Assurance | Oct 14 to Nov 25 | Jan 25 to Jul 3 | Feb 21 | N/A | Mar 1 to Mar 2 | Jul 3 (Thursday) |
Jul 18 | Jul 22 | Closed |
Extended Summer 2025
Session | Registration Period | Module Dates | Withdrawal Deadline | Orientation Workshop | Module Workshop | Exam Date | Results Release | Appeals Deadline | Register |
---|---|---|---|---|---|---|---|---|---|
Core 1 | Apr 14 to May 26 | Jul 26 to Dec 18 | Aug 22 | Jul 26 to Jul 27 | Oct 25 to Oct 26 | Dec 18 (Thursday) |
Jan 16 | Jan 20 | Upcoming |
Core 2 | Apr 14 to May 26 | Jul 26 to Dec 17 | Aug 22 | N/A | Nov 8 to Nov 9 | Dec 17 (Wednesday) |
Jan 16 | Jan 20 | Upcoming |
Assurance | Apr 14 to May 26 | Jul 26 to Dec 18 | Aug 22 | N/A | Aug 30 to Aug 31 | Dec 18 (Thursday) |
Jan 16 | Jan 20 | Upcoming |
Taxation | Apr 14 to May 26 | Jul 26 to Dec 17 | Aug 22 | N/A | Oct 4 to Oct 5 | Dec 17 (Wednesday) |
Jan 16 | Jan 20 | Upcoming |
Finance | Apr 14 to May 26 | Jul 26 to Dec 17 | Aug 22 | N/A | Sep 20 to Sep 21 | Dec 17 (Wednesday) |
Jan 16 | Jan 20 | Upcoming |
Winter 2025
Session | Registration Period | Module Dates | Withdrawal Deadline | Orientation Workshop | Module Workshop | Exam Date | Results Release | Appeals Deadline | Register |
---|---|---|---|---|---|---|---|---|---|
Core 1 | Oct 14 to Nov 25 | Jan 25 to Mar 27 | Feb 21 | Jan 25 to Jan 26 | Mar 1 to Mar 2 | Mar 27 (Thursday) |
April 11 | Apr 15 | Closed |
Core 2 | Oct 14 to Nov 25 | Jan 25 to Mar 26 | Feb 21 | N/A | Mar 8 to Mar 9 | Mar 26 (Wednesday) |
April 11 | Apr 15 | Closed |
Performance Management | Oct 14 to Nov 25 | Jan 25 to Mar 27 | Feb 21 | N/A | Mar 1 to Mar 2 | Mar 27 (Thursday) |
April 11 | Apr 15 | Closed |
Assurance | Oct 14 to Nov 25 | Jan 25 to Mar 27 | Feb 21 | N/A | Feb 8 to Feb 9 | Mar 27 (Thursday) |
April 11 | Apr 15 | Closed |
Finance | Oct 14 to Nov 25 | Jan 25 to Mar 26 | Feb 21 | N/A | Feb 15 to Feb 16 | Mar 26 (Wednesday) |
April 11 | Apr 15 | Closed |
Taxation | Oct 14 to Nov 25 | Jan 25 to Mar 26 | Feb 21 | N/A | Feb 22 to Feb 23 | Mar 26 (Wednesday) |
April 11 | Apr 15 | Closed |
Capstone 1 | Oct 14 to Nov 25 | Jan 25 to Mar 27 | Feb 21 | N/A | Jan 25 to Jan 26 | Presentations Mar 21 to Mar 27 |
Mar 28 | N/A | Closed |
Spring 2025
Session | Registration Period | Module Dates | Withdrawal Deadline | Orientation Workshop | Module Workshop | Exam Date | Results Release | Appeals Deadline | Register |
---|---|---|---|---|---|---|---|---|---|
Core 1 | Jan 20 to Mar 3 | May 3 to Jul 3 | May 30 | May 3 to May 4 | Jun 7 to Jun 8 | Jul 3 (Thursday) |
Jul 18 | Jul 22 | Closed |
Core 2 | Jan 20 to Mar 3 | May 3 to Jul 4 | May 30 | N/A | Jun 14 to Jun 15 | Jul 4 (Friday) |
Jul 18 | Jul 22 | Closed |
Performance Management | Jan 20 to Mar 3 | May 3 to Jul 3 | May 30 | N/A | May 10 to May 11 | Jul 3 (Thursday) |
Jul 18 | Jul 22 | Closed |
Assurance | Jan 20 to Mar 3 | May 3 to Jul 3 | May 30 | N/A | May 17 to May 18 | Jul 3 (Thursday) |
Jul 18 | Jul 22 | Closed |
Finance | Jan 20 to Mar 3 | May 3 to Jul 4 | May 30 | N/A | May 24 to May 25 | Jul 4 (Friday) |
Jul 18 | Jul 22 | Closed |
Taxation | Jan 20 to Mar 3 | May 3 to Jul 4 | May 30 | N/A | May 31 to Jun 1 | Jul 4 (Friday) |
Jul 18 | Jul 22 | Closed |
Capstone 1 | Jan 27 to Mar 10 | May 10 to Jul 11 | Jun 6 | N/A | May 10 to May 11 | Presentations Jul 4 to Jul 10 |
Jul 11 | N/A | Closed |
Capstone 2 | Dec 16 to Feb 3 | Mar 29 to May 16 | Apr 25 | N/A | Mar 29 to Mar 30 | N/A | TBD | N/A | Closed |
CFE | Dec 15 to Feb 3 | N/A | May 20 | N/A | N/A | May 21 to May 23 | Aug 8 | Aug 22 | Closed |
Summer 2025
Session | Registration Period | Module Dates | Withdrawal Deadline | Orientation Workshop | Module Workshop | Exam Date | Results Release | Appeals Deadline | Register |
---|---|---|---|---|---|---|---|---|---|
Core 1 | Apr 14 to May 26 | Jul 26 to Sep 25 | Aug 22 | Jul 26 to Jul 27 | Aug 30 to Aug 31 | Sep 25 (Thursday) |
Oct 10 | Oct 14 | Upcoming |
Core 2 | Apr 14 to May 26 | Jul 26 to Sep 24 | Aug 22 | N/A | Sep 6 to Sep 7 | Sep 24 (Wednesday) |
Oct 10 | Oct 14 | Upcoming |
Performance Management | Apr 14 to May 26 | Jul 26 to Sep 25 | Aug 22 | N/A | Aug 2 to Aug 3 | Sep 25 (Thursday) |
Oct 10 | Oct 14 | Upcoming |
Assurance | Apr 14 to May 26 | Jul 26 to Sep 25 | Aug 22 | N/A | Aug 9 to Aug 10 | Sep 25 (Thursday) |
Oct 10 | Oct 14 | Upcoming |
Finance | Apr 14 to May 26 | Jul 26 to Sep 24 | Aug 22 | N/A | Aug 16 to Aug 17 | Sep 24 (Wednesday) |
Oct 10 | Oct 14 | Upcoming |
Taxation | Apr 14 to May 26 | Jul 26 to Sep 24 | Aug 22 | N/A | Aug 23 to Aug 24 | Sep 24 (Wednesday) |
Oct 10 | Oct 14 | Upcoming |
Capstone 2 | Apr 7 to May 20 | Jul 19 to Sep 5 | Aug 15 | N/A | Jul 19 to Jul 20 | N/A | TBD | N/A | Upcoming |
Fall 2025
Session | Registration Period | Module Dates | Withdrawal Deadline | Orientation Workshop | Module Workshop | Exam Date | Results Release | Appeals Deadline | Register |
---|---|---|---|---|---|---|---|---|---|
Core 1 | Jul 7 to Aug 18 | Oct 18 to Dec 18 | Nov 14 | Oct 18 to Oct 19 | Nov 22 to Nov 23 | Dec 18 (Thursday) |
Jan 16 | Jan 20 | Upcoming |
Core 2 | Jul 7 to Aug 18 | Oct 18 to Dec 17 | Nov 14 | N/A | Nov 29 to Nov 30 | Dec 17 (Wednesday) |
Jan 16 | Jan 20 | Upcoming |
Performance Management | Jul 7 to Aug 18 | Oct 18 to Dec 18 | Nov 14 | N/A | Oct 25 to Oct 26 | Dec 18 (Thursday) |
Jan 16 | Jan 20 | Upcoming |
Assurance | Jul 7 to Aug 18 | Oct 18 to Dec 18 | Nov 14 | N/A | Nov 1 to Nov 2 | Dec 18 (Thursday) |
Jan 16 | Jan 20 | Upcoming |
Finance | Jul 7 to Aug 18 | Oct 18 to Dec 17 | Nov 14 | N/A | Nov 8 to Nov 9 | Dec 17 (Wednesday) |
Jan 16 | Jan 20 | Upcoming |
Taxation | Jul 7 to Aug 18 | Oct 18 to Dec 17 | Nov 14 | N/A | Nov 15 to Nov 16 | Dec 17 (Wednesday) |
Jan 16 | Jan 20 | Upcoming |
CFE | Apr 7 to May 26 | N/A | Sep 8 | N/A | N/A | Sep 9 to Sep 11 | Nov 28 | Dec 12 | Upcoming |
Winter 2026
Session | Registration Period | Module Dates | Withdrawal Deadline | Orientation Workshop | Module Workshop | Exam Date | Results Release | Appeals Deadline | Register |
---|---|---|---|---|---|---|---|---|---|
Core 1 | Oct 13 to Nov 24 | Jan 24 | Feb 27 | Jan 24 to Jan 25 | Feb 28 to Mar 1 | Mar 26 (Thursday) |
Apr 10 | Apr 14 | Upcoming |
Core 2 | Oct 13 to Nov 24 | Jan 24 to Mar 25 | Feb 27 | N/A | Mar 7 to Mar 8 | Mar 25 (Wednesday) |
Apr 10 | Apr 14 | Upcoming |
Performance Management | Oct 13 to Nov 24 | Jan 24 to Mar 26 | Feb 27 | N/A | Jan 31 to Feb 1 | Mar 26 (Thursday) |
Apr 10 | Apr 14 | Upcoming |
Assurance | Oct 13 to Nov 24 | Jan 24 to Mar 26 | Feb 27 | N/A | Feb 7 to Feb 8 | Mar 26 (Thursday) |
Apr 10 | Apr 14 | Upcoming |
Finance | Oct 13 to Nov 24 | Jan 24 to Mar 25 | Feb 27 | N/A | Feb 14 to Feb 15 | Mar 25 (Wednesday) |
Apr 10 | Apr 14 | Upcoming |
Taxation | Oct 13 to Nov 24 | Jan 24 to Mar 25 | Feb 27 | N/A | Feb 21 to Feb 22 | Mar 25 (Wednesday) |
Apr 10 | Apr 14 | Upcoming |
Capstone 1 | Oct 13 to Dec 1 | Jan 24 | Feb 27 | N/A | Jan 24 to Jan 25 | Presentation Dates TBD |
Mar 27 | N/A | Upcoming |
Spring 2026
Session | Registration Period | Module Dates | Withdrawal Deadline | Orientation Workshop | Module Workshop | Exam Date | Results Release | Appeals Deadline | Register |
---|---|---|---|---|---|---|---|---|---|
Core 1 | Jan 19 to Mar 2 | May 2 to Jun 30 | Jun 5 | May 2 to May 3 | Jun 6 to Jun 7 | Jun 30 (Tuesday) |
Jul 17 | Jul 21 | Upcoming |
Core 2 | Jan 19 to Mar 2 | May 2 to Jun 29 | Jun 5 | N/A | Jun 13 to Jun 14 | Jun 29 (Monday) |
Jul 17 | Jul 21 | Upcoming |
Performance Management | Jan 19 to Mar 2 | May 2 to Jun 30 | Jun 5 | N/A | May 9 to May 10 | Jun 30 (Tuesday) |
Jul 17 | Jul 21 | Upcoming |
Assurance | Jan 19 to Mar 2 | May 2 to Jun 30 | Jun 5 | N/A | May 16 to May 17 | Jun 30 (Tuesday) |
Jul 17 | Jul 21 | Upcoming |
Finance | Jan 19 to Mar 2 | May 2 to Jun 29 | Jun 5 | N/A | May 23 to May 24 | Jun 29 (Monday) |
Jul 17 | Jul 21 | Upcoming |
Taxation | Jan 19 to Mar 2 | May 2 to Jun 29 | Jun 5 | N/A | May 30 to May 31 | Jun 29 (Monday) |
Jul 17 | Jul 21 | Upcoming |
Capstone 1 | Jan 19 to Mar 9 | May 9 | Jun 12 | N/A | May 9 to May 10 | Presentation Dates TBD |
Jul 10 | N/A | Upcoming |
Capstone 2 | Dec 29 to Feb 9 | Apr 11 | May 15 | N/A | Apr 11 to Apr 12 | N/A | TBD | N/A | Upcoming |
Summer 2026
Session | Registration Period | Module Dates | Withdrawal Deadline | Orientation Workshop | Module Workshop | Exam Date | Results Release | Appeals Deadline | Register |
---|---|---|---|---|---|---|---|---|---|
Core 1 | Apr 13 to May 25 | Jul 25 to Sep 24 | Aug 28 | Jul 25 to Jul 26 | Aug 29 to Aug 30 | Sep 24 (Thursday) |
Oct 9 | Oct 13 | Upcoming |
Core 2 | Apr 13 to May 25 | Jul 25 to Sep 23 | Aug 28 | N/A | Sep 5 to Sep 6 | Sep 23 (Wednesday) |
Oct 9 | Oct 13 | Upcoming |
Performance Management | Apr 13 to May 25 | Jul 25 to Sep 24 | Aug 28 | N/A | Aug 1 to Aug 2 | Sep 24 (Thursday) |
Oct 9 | Oct 13 | Upcoming |
Assurance | Apr 13 to May 25 | Jul 25 to Sep 24 | Aug 28 | N/A | Aug 8 to Aug 9 | Sep 24 (Thursday) |
Oct 9 | Oct 13 | Upcoming |
Finance | Apr 13 to May 25 | Jul 25 to Sep 23 | Aug 28 | N/A | Aug 15 to Aug 16 | Sep 23 (Wednesday) |
Oct 9 | Oct 13 | Upcoming |
Taxation | Apr 13 to May 25 | Jul 25 to Sep 23 | Aug 28 | N/A | Aug 22 to Aug 23 | Sep 23 (Wednesday) |
Oct 9 | Oct 13 | Upcoming |
Capstone 2 | Apr 13 to May 25 | Jul 25 | Aug 28 | N/A | Jul 25 to Jul 26 | N/A | TBD | N/A | Upcoming |
CFE | Dec 29 to Feb 16 | Jun 2 | Jun 1 | N/A | N/A | Jun 2 to Jun 4 | TBD | TBD | Upcoming |
Fall 2026
Session | Registration Period | Module Dates | Withdrawal Deadline | Orientation Workshop | Module Workshop | Exam Date | Results Release | Appeals Deadline | Register |
---|---|---|---|---|---|---|---|---|---|
Core 1 | Jul 6 to Aug 17 | Oct 17 to Dec 17 | Nov 20 | Oct 17 to Oct 18 | Nov 21 to Nov 22 | Dec 17 (Thursday) |
Jan 15 | Jan 19 | Upcoming |
Core 2 | Jul 6 to Aug 17 | Oct 17 to Dec 16 | Nov 20 | N/A | Nov 28 to Nov 29 | Dec 16 (Wednesday) |
Jan 15 | Jan 19 | Upcoming |
Performance Management | Jul 6 to Aug 17 | Oct 17 to Dec 17 | Nov 20 | N/A | Oct 24 to Oct 25 | Dec 17 (Thursday) |
Jan 15 | Jan 19 | Upcoming |
Assurance | Jul 6 to Aug 17 | Oct 17 to Dec 17 | Nov 20 | N/A | Oct 31 to Nov 1 | Dec 17 (Thursday) |
Jan 15 | Jan 19 | Upcoming |
Finance | Jul 6 to Aug 17 | Oct 17 to Dec 16 | Nov 20 | N/A | Nov 7 to Nov 8 | Dec 16 (Wednesday) |
Jan 15 | Jan 19 | Upcoming |
Taxation | Jul 6 to Aug 17 | Oct 17 to Dec 16 | Nov 20 | N/A | Nov 14 to Nov 15 | Dec 16 (Wednesday) |
Jan 15 | Jan 19 | Upcoming |
CFE | Apr 13 to Jun 1 | Sep 15 | Sep 14 | N/A | N/A | Sep 15 to Sep 17 | TBD | TBD | Upcoming |