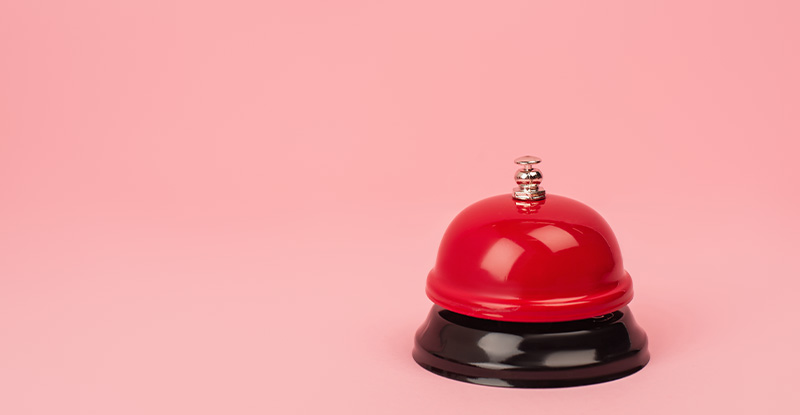
Photo credit: IgorVetushko/Depositphotos.com
CPA Western School of Business provides reasonable one-time and ongoing exam accommodations to learners for a variety of reasons. The purpose of this post is to outline the general timeline of when notable items occur within the exam accommodations process for learners.
At least 10 weeks* before the exam date
- Submit your accommodation request, including all supporting documentation for review.
- More information on requests and required documentation can be found here.
8 weeks before the exam date
- The CPA profession's National Accommodations Advisory Panel meets monthly to review learner accommodation requests.
- Once all documentation is received, your request will be reviewed at the next scheduled panel meeting and a decision will be made.
6 weeks before the exam date
- CPAWSB will communicate the outcome of your accommodation request to you via email.
- CPAWSB will work behind the scenes to ensure your approved accommodation is effectively implemented for your upcoming exam.
2 weeks before the date
- CPAWSB will email you to confirm your exam accommodation details, such as exam timing, location (when exams take place at in-person exam centres), and any other applicable instructions.
* Accommodation requests may be accepted after the deadline due to extenuating circumstances, such as an accident. In these cases, submit the request as soon as possible, so that requests can be reviewed and effectively implemented if approved.
Please send questions and submit completed accommodation request forms and supporting documentation to [email protected].
For information on when an exam accommodation may be available to learners in CPA programs, please see this previous blog post.